1. Introduction
Rapid urbanization has been taking place worldwide. The number of world’s cities with a population over one million increased from 371 in 2000 to 548 in 2018 (UN, 2019). Additionally, 33 megacities, including New York, Seoul, Shanghai, London, Tokyo, and Sao Paulo, have developed high density, with populations exceeding 10 million (United Nations, 2019). High-density cities are known to induce physical problems, such as the urban heat island effect, air pollution, and flood issues, spatial access inequity (Liang et al., 2016) and public service disparities (Wolch et al., 2014). Green spaces, including urban forests and public parks, have been proposed as components of urban development to address these issues. These green spaces are also recognized as infrastructures that enhance a city’s environmental comfort (Kim and Lee, 2016; Rafael et al., 2018; Teshnehdel et al., 2020) and promote the development of sustainable communities (Kramer, 2014).
Newly developing cities are creating urban parks based on careful urban planning. However, older high-density cities that emerged organically often have incomplete institutions for urban parks or lack physically appropriate sites for parks because the rapid population increase was not anticipated when the city was first built. It is difficult to secure new spaces in an old city that has already been fully developed. For this reason, there is a limit to adding green infrastructures, such as urban public parks (Hwang, 2016).
Therefore, instead of developing new physical green infrastructures, current efforts focus either on expanding the benefits of park use to more city residents by considering the parks from the perspective of equity or enhancing park users’ satisfaction by helping them utilize the spaces more effectively and efficiently. Previous works on urban park equity have mainly discussed spatial equity issues (Lucy, 1988) and evaluated park areas based on accessibility concepts (Talen and Anselin, 1998; Oh and Jeong, 2007; Boone et al., 2009; Sister et al., 2010). Studies on user satisfaction with parks have investigated factors that increase park users’ satisfaction, using surveys and on-site observations (Schipperijn et al., 2013; Mak and Jim, 2019). And there was expanding the benefits of park use by to revitalize park use (i.e., mixed land use [Chen et al., 2018; Bahriny and Bell, 2020; Fry et al., 2021], expanding park programs).
However, studies focusing on accessibility concepts and the expansion of the benefits of park usage have neglected the viewpoint of the spatial distribution of park users, which is important for planning spatial policy or park operations. Some previous researchers analyzed the spatial distribution information produced by various media, such as social networking service (SNS) data (Chen et al., 2018; Kumar et al., 2018) or review data (Park et al., 2017). Nevertheless, these datasets, such as SNS or review data, did not offer reliable information on where park users came from. As a result, these studies have not provided spatial policy solutions for getting more city residents to use urban parks.
This study investigates the distribution of urban park users to fill a gap in existing research where it is difficult to know where they are coming from, so that visitors can use urban parks more effectively and efficiently. The study will utilize data to identify user hotspots within urban parks and investigate the extent of their impact on the park. It also explores the relationship between the urban context (e.g., neighborhood land uses, traffic conditions) and the extent of park impacts. The aim of this sutdy is to provide practical implications that can be utilized in the planning and management of parks.
2. Materials and Methods
South Korea has the highest smartphone ownership rate (Poushter et al., 2018). According to the Korean Media Panel Survey conducted by the Korea Information Society Development Institute (KISDI), Seoul’s mobile phone ownership rate in 2018 was 97.0% (94.0% nationwide). As a survey, mobile phone ownership rate in Seoul is higher than in other regions, and Seoul Metropolitan Government was also using it. Therefore, Seoul was selected as the study site. Due to the limitations of the available mobile signal data, the research was conducted in Seongdong-gu, one of the 25 boroughs in Seoul, which is home to the ‘Metropolitan Area Neighborhood Park’ and features a wide-area park known as Seoul Forest Park. In addition, Seongdong-gu is an area where parks utilizing mountainous areas and parks centered on neighborhoods exist. So, we have selected Seongdong-gu, Seoul, as a study area. Five urban public parks in Seongdong-gu were selected for the study. The five selected parks were based on the mobile signaling data legally provided by the Seoul Metropolitan Government for research purposes. Within Seongdong-gu, each park was selected based on different land use and physical characteristics, such as area and slope. Each location of the five urban parks selected for this study is marked in Figure 1. The individual parks have the following characteristics Seoul Forest (P1) was created in 2005 and the park is not managed directly by the Seoul Metropolitan Government, but through an external partnership agreement. Seoul Government is only in charge of hardware maintenance, so the park is managed without regard to administrative divisions. The park has the most park users, and the area around the park is a mix of residential and commercial facilities. Eungbong Park (P2) was created in 2003 and features a view of the Han River and various convenience and sports facilities (tennis courts, gateball courts, beteminton courts, multipurpose stadiums, etc.). The park’s facilities are actively used and has many users. Seongsu Park (P3) was created in 1998 and is a park built on a former factory site. Currently, it is surrounded by dense commercial areas. Dokseodang Park (P4) and Daehyunsan Park (P5) are parks that utilize mountainous areas, unlike existing parks built on flat land. P4 is surrounded by apartments except for the entrance and P5 has walking trails, sports facilities, a nature learning center, and a youth reading room (Figure 2).
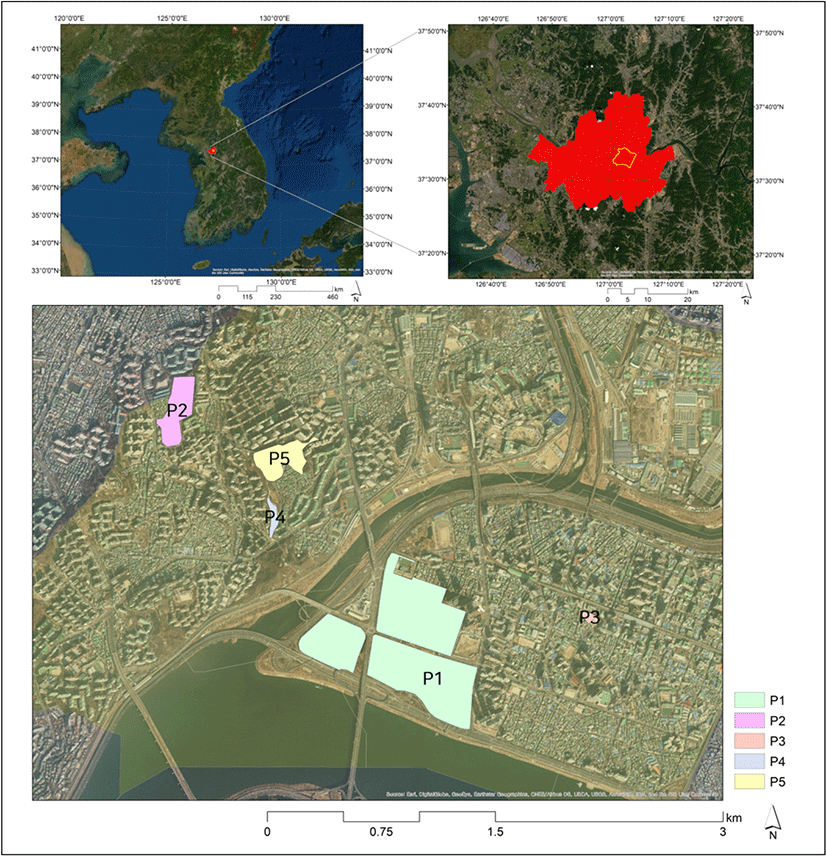
Mobile signaling data are used to identify users’ origins and the extent of park impact (Zhai et al., 2018; Guo et al., 2019; Guan et al., 2020; 2021). Signaling data and administrative and park boundaries were used to analyze the extent of park impact. Land use, physical properties, population, and transportation data were utilized to understand the correlation between park impact boundaries and urban planning factors. Park boundary data were created using Google Maps (Table 1, Figure 3).
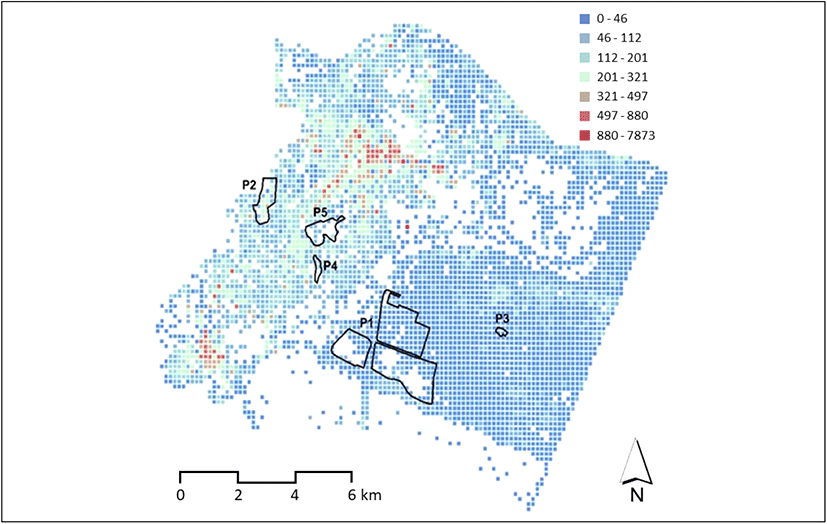
Floating population data aggregates the movement of inflow and outflow based on mobile phone use and user address. It can be used to examine population distributions related to spatial accessibility analysis (Claudia et al., 2022). The floating population data used in this study were collected by SK Telecom (SKT), the largest telecommunications company in Korea. They generated statistical data in units of pCell (50m by 50m unit) by utilizing BTS (Base Transceiver Stations) information accumulated with signal data and call data records (traffic information such as call, message, and mobile data). This data represented the active population, measuring the number of people who stayed in a particular unit for more than 15 minutes. Used data was the average floating population data corresponding to one hour of the floating population per day. The data were received by the Seoul Metropolitan Government Big Data Campus. They were originally provided by SK Telecom and limited to Seoul Metropolitan City. The data were statistically processed on a monthly basis and had a structured format including information such as date, x-y coordinates, sex, age, origin code, and the number of floating population (Table 2).
The data were processed to extract the information for this study (Figure 4). Five groups were created based on the selected parks, and unnecessary information, such as gender code, was deleted. The demographic statistics of park visitors were derived from the processed dataset, and inflow distribution maps were generated with the origin codes.
This study proceeded as shown in Figure 5. The analysis of inflow population from each administrative unit and inflow distribution was conducted using the floating population data for each park. Hot spot analysis was used to identify local spatial autocorrelations and understand the service range of the ecosystem (Wu et al., 2013; Baumeister et al., 2020). If a spatial correlation represented as ‘Getis-Ord General G’ and ‘Moran’s I’ was derived through global spatial correlation analysis, a local spatial autocorrelation pattern was created (Getis and Ord, 2010). To identify local spatial autocorrelation, the hot spot analysis of ArcMap 10.3 was applied. User hot spot areas were identified with a 90% confidence level.
Physical characteristics of the five parks, the number of park visitors, and land use of the surrounding areas were identified for the spatial statistics analysis. To analyze the physical characteristics of the parks, such as the average slope and size, a digital elevation model (DEM) was used. Surrounding areas were defined within a 100 m buffer from the edges of the park. Statistical analysis was conducted on the ratio of land use, population, and population density. For land use, areas designated as roads, waterfronts, or rivers were excluded.
Based on user hot spot analysis and spatial statistics analysis, additional analysis was carried out to shed light on the relationship between park use and the urban environment surrounding the park. The relationship between park use and the urban environment was analysed and discussed based on existing information about the park, such as information on the surrounding environment of urban parks and survey information on park users.
3. Result and Discussion
The results of user hot spot analysis confirmed that, among the five parks, P1 and P3 had wider geographical influence. For instance, 70 administrative units for P1 were user inflow hot spots. For P2 and P3, these numbers were 47 and 67 administrative units, respectively. P4 and P5 had the same number of user inflow hot spots, 45 (Table 3). It appeared that P1 was used mainly by residents of Seongdong-gu and the neighboring boroughs of Seongdong-gu, Gwangjin-gu, and Jung-gu, as well as a part of Dongdaemun-gu. Many visitors came from Gangnam-gu, south of the Han River. P2’s core hot spot areas covered mainly Seongdong-gu and a part of Jung-gu, Gwangjin-gu, and Dongdaemun-gu. P3 was used from Gwangjin-gu in the east. P4 and P5 showed similar inflow administrative units, mainly from Seongdong-gu and the neighboring boroughs of Seongdong-gu, Gwangjin-gu, and Jung-gu, as well as a part of Dongdaemun-gu. The areas close to the parks had a higher utilization level, but user hot spots appeared in P1 from Gangnam-gu and P3 from the entire Gwangjin-gu (Figure 6).
Park | Core hot spot area (administrative unit) |
---|---|
P1 | 70 |
P2 | 47 |
P3 | 67 |
P4 | 45 |
P5 | 45 |
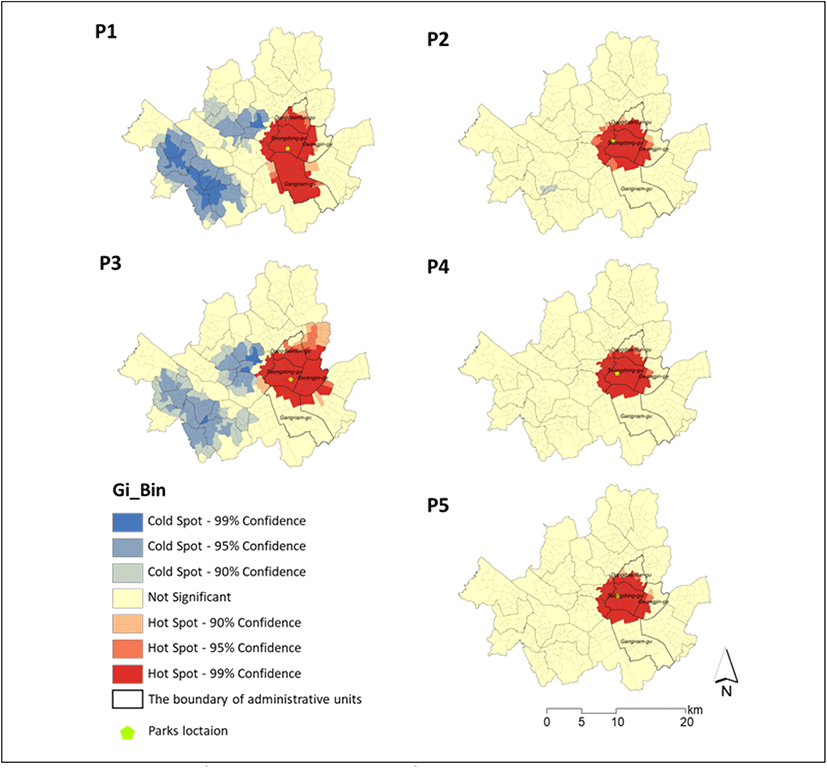
Global spatial autocorrelation analysis was conducted to verify the spatial autocorrelation derived from the hot spot analysis. Both ‘Getis-Ord General G’ and ‘Moran’s I’ showed the autocorrelation, meaning that the user hot spots were reliable. P1 and P3 had a lower global spatial autocorrelation than P2, P4, and P5. This is consistent with the finding that P1 and P3 had many hot spots (Table 4).
Park | Z-score (Getis-Ord General G) | Moran’s I |
---|---|---|
P1 | 20.23 | 0.763 |
P2 | 39.87 | 0.8864 |
P3 | 21.61 | 0.7495 |
P4 | 38 | 0.8184 |
P5 | 38.73 | 0.8136 |
In prior studies, the service range of urban parks was defined as the area where potential users existed, and the service range was proposed based on the effort required, such as distance or time, for users to reach the park (Oh and Jeong, 2007; Boone et al., 2009; Sister et al., 2010). However, by using mobile signaling data to identify the distrubution of park users and conducting hotspot analysis, it is possible to derive the extent of a park’s impact. This approach allows for overcoming the limitations of field surveys and observational studies, which often cannot capture daily user inflow patterns or information across multiple urban parks.
The analysis of the relationship between the core hot spots of each park and the surrounding land use showed that parks with a high ratio of commercial areas had a wider range of influence than parks that did not. P2, P4, and P5, located in residential areas, showed core hot spots around the park. P1, located in a mixed-use area, and P3, located in a commercial area, had wider core hot spots. The residential ratios of P2, P4, and P5 were significantly higher than those of P1 and P3. The land use pattern of the area surrounding P1 showed a mix of residential, commercial, industrial, government, and vacant lots. It was assumed that P3 had a higher commercial use ratio because the park was relatively smaller than others and located in a dense commercial area (Table 5).
P2, P4, and P5, located in residential areas, had narrower core hot spots, perhaps because people could visit them frequently due to the convenient location near home (Cohen et al., 2007; Liu et al., 2017). P1 and P3, located in commercial or mixed-use areas, had wider core hot spots because visitors who were employees in the commercial areas were often park users (Choi and Kim, 2016) (Figure 7). According to Guo et al. (2019), the more employment places there were located near the park, the stronger the effect was on weekdays. Therefore, the higher commercial ratio in the area near the park was correlated to wider core hot spots.
P1 also had wider core hot spots during the week. However, the difference between weekdays and weekends was not significant (Figure 8) due to the different types of park users. While P3 visitors were mainly workers in the commercial areas nearby, P1 had a high percentage of users who visited the park with families and friends (Kim et al., 2017). In addition, P1’s pattern of core hot spots extended in a north-south direction because the accessibility factor to P1 was high (Figure 9). The correlation analysis between the inflow population by administrative units and accessibility factors (i.e., distance from the park nearby, distance from adjacent subway stations) showed that park users tended to visit more as they got closer to the subway station near their home and use more of P1 as they lived far from similar parks with various open spaces (Table 6). The more convenient accessibility to mass transportation facilities was, the more the park was used (Muftuler et al., 2011; Chow er al., 2016; Li et al., 2017), and the farther people came to use the park (Neuvonen et al., 2007; Sugiyama et al., 2010; Cao et al., 2015). The analysis of visitors’ means of transportation for P1 showed that the park’s accessibility on foot was lower than for the other parks (average = 57.0%, P1 = 18.7%), and the mass transportation usage rate was relatively higher (average = 24.8%, Seoul Forest = 42.9%) (Kim et al., 2017).
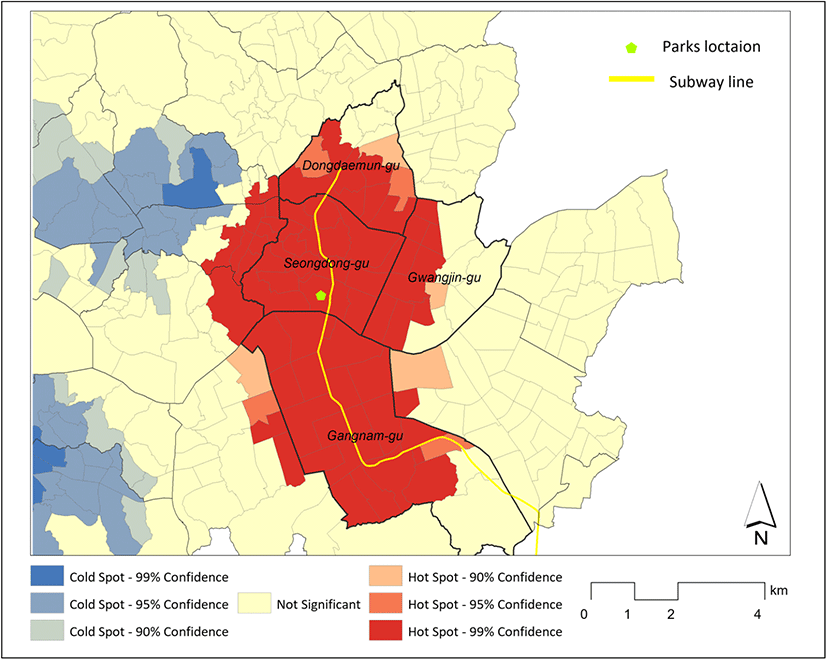
Park | Distance from subway station | Distance from similar parks |
---|---|---|
Park user | −0.553* | 0.260** |
The findings showed that the core hot spots of urban parks differed depending on the type of urban park surroundings (i.e., residential, commercial, or mixed-use). The parks in residential areas showed constant user hot spots on weekends and weekdays. In contrast, those in commercial areas were widely used on weekdays, where the influence of commuting was high. It has been explained that people use parks to get out of the crowd (Chiesura, 2004). However, the use of commercial areas and the use of parks were compatible in this study. In other research, a park was actively used if located in a mixed-use area (Parra et al., 2010; Zhu et al., 2020; Fry et al., 2021). It was also found that parks in mixed-use areas tended to generate motivation to attract users from far away (Wu et al., 2018). And transportation has played a positive role in expanding the park’s service area.
Table 7 presents the statistics of park users for the five selected parks. The average number of users on weekdays and weekends was 22,589 for P1, 20,856 for P2, 437 for P3, 9,911 for P4, and 2,761 for P5. While P1, P2, and P3 had relatively flat terrain, P4 and P5 had steep slopes. P1, P2, and P5 were larger parks, but P3 and P5 were small parks. P1 and P2 had not only flat terrain but also large open spaces. Conversely, P3 lacked large open spaces due to its small size. P4 was large but without much open space because it was in a mountainous area. P5 had a steep slope, making it difficult to have large open spaces that could attract more visitors.
The greater number of park visitors recorded for P1 and P2 may be attributed to physical characteristics, such as open spaces. This accords with the results of previous studies, which found that parks on flat terrain can satisfy the needs of more diverse park usage than parks with steep slopes (Schipperijn et al., 2010; Wan et al., 2020). In addition, the finding that larger parks had more visitors (Muftuler et al., 2011; Wolch et al., 2014; Chow et al., 2016) is in line with Wan et al.’s (2020) analysis that wide-open spaces or various facilities satisfy the needs of various usage.
This study confirmed that larger parks with various park programs had wider hot spots. This tendency was also shown in research showing that large-scale urban parks encourage users’ participation (Chow et al., 2016; Muftuler et al., 2011). Large-scale urban parks could provide more variety of activities (Schipperijn et al., 2010; Hamstead et al., 2018). As suggested in most studies (Neuvonen et al., 2007; Sugiyama et al., 2010; Wang et al., 2015; Hamstead et al., 2018; Zhu et al., 2020).
It was confirmed that the usage range of urban parks differed depending on the characteristics of the park, characteristics of the surrounding area, and availability of public transportation. Cities change their demographic characteristics over time, and it is important to understand the impact these demographic changes have on parks, which are part of the city infrastructure. This research identified the user hot spots by age and its characteristics of using the park.
The results confirmed that the working-age population that visited the park came from farther away than the dependent population. Other studies have argued that the working-age population has a wider activity range than the dependent population (Neuvonen et al., 2007; Chow et al., 2016). As this tendency is prominent in parks surrounded by commercial and industrial areas, it is believed that commercial and industrial facilities have affected the influx of working-age people into the park. In other words, the inflow of the working-age population generates wider hot spots. Guo et al. (2019) found a wider impact range during the weekdays in places with more workplaces. In this study, all five parks showed a wider core hot spot on weekdays than on weekends, but the difference between P1 and P3 was remarkable. Unlike P2, P4, and P5, which were in the residential areas, P1 and P3, in commercial and mixed-use areas, showed a low ratio of the dependent population among their visitors (Figure 10).
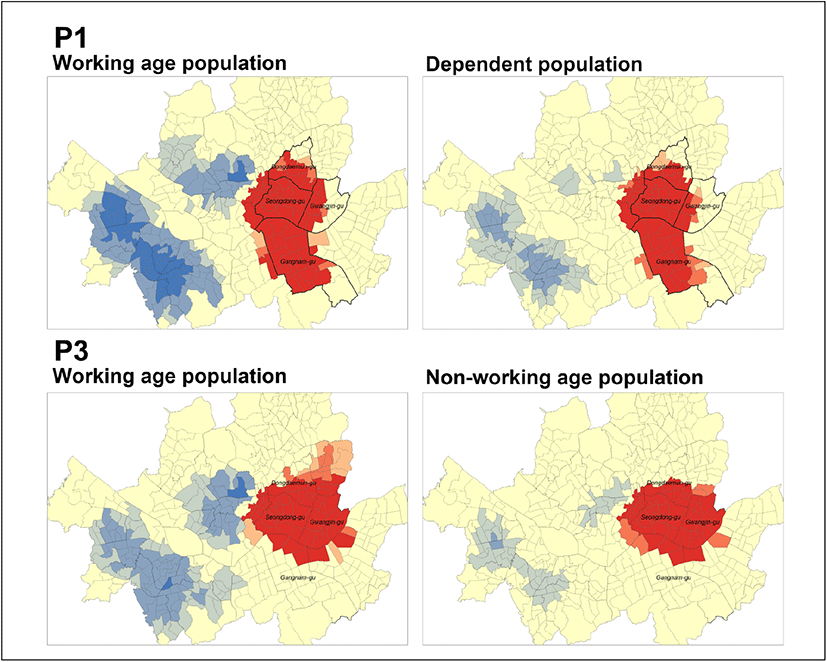
Another reason the distribution of users by age is different could be differences in the purpose of park use between the working and nonworking population. The results of a survey conducted by the Seoul Metropolitan Government in 2017 showed that the purpose of park use differed by users’ age (Kim et al., 2017). Those aged 50–60 years (50s = 88.8%, 60s or older = 94.2%) visited the park to walk, rest, and exercise. Meanwhile, those in their 20s (66.6%) tended to report using the park as a meeting place (22.9%, average = 8.6%). Those aged 30–40 years used the park as a learning place for their children (30s = 20.6%, 40s and over = 12.1%), higher than the average (6.4%). For respondents in their 20s and 30–40s, the percentage of those who used the park for walking, rest, and exercise was low. In other words, the purpose of park visits differed by age; those in the working-age population showed various usage behaviors distinct from those of the dependent population. Schipperijn et al. (2010) mentioned that park users may travel some distance to reach a park because they choose parks that fulfill their various needs. In this research, the park users with various purposes were mainly those in the working-age population.
Additionally, the movement range of the dependent population was limited (Reyes et al., 2014). According to a survey by the Seoul Metropolitan Government, the percentage of the population aged 60s or older was higher than average (57%), and this group reported that walking (78.5%) was their main means of transportation. The survey similarly reported that the percentage of people who said walking was their main means of transportation was higher for people in their 50s and 60s than younger age groups (Kim et al., 2017).
After looking at the distribution of park user inflow, it is necessary to identify other complex factors affecting user hot spots, such as land use and transportation infrastructure, besides the distance and time to commute to the park, as these were considered in previous studies and urban park planning. This study found that parks in commercial, industrial, and mixed-use areas had a wider user inflow than parks located in residential areas. Furthermore, park user inflow was affected by the transportation infrastructure.
Public parks are essential green infrastructure in cities. More parks are needed; however, it is difficult to find space to develop new parks in dense urban areas. To solve this problem, it is necessary to expand the public transportation system or link the park to a commercial area nearby because neighborhood revitalization could be achieved when the park interacts with the commercial area (Park and Kim, 2019).
Then, concerning park management, it is important to understand the user distribution and its range of impact. Because the main body that manages urban parks is the local government, the parks are managed according to the physical boundaries of administrative districts. However, this paper showed that many park users came from beyond one administrative district. To manage the park, including visitors who live nearby and those who come from a distance, it is necessary to consider an individual management system for each park. For self-management, it is necessary to identify the distribution of park users and derive core hot spots.
4. Conclusions
In this paper, the distribution of user inflow was identified using mobile signaling data, and user hot spots were derived through the inflow distribution. The analysis of the relationship between the hot spots for park use and the urban environment showed which urban parks had wider core hot spots. It was confirmed that the distribution of park users differed according to land use. Specifically, residential areas showed a concentrated hot spot centered on the target park, and parks in the commercial area showed wider core hot spots. Physical characteristics also influenced park use.
The findings suggest it is more effective to either create new urban parks in high-density cities or use existing parks in conjunction with commercial areas instead of residential areas. Moreover, it helps people to use the park when it is connected to the public transportation system. Parks with gentle slopes attract more visitors because this allows them to be used for various activities.
And this study utilized mobile signaling data, it is possible to change the park management by target users, especially inflow population. In addition, it is expected that research on improving the public transportation system around the park will be possible by confirming the accessibility of the inflow of population.
Furthermore, this study suggests urban planners with a different perspective and approach on planning or management. Urban parks were designated by the zoning process, as a necessary space for neighborhoods. So, the target of user of the park has limited the neighborhood living area, but according to the results of this study, the user is not limited to the administrative district. The characteristics of inflow users that can be confirmed through data can also affect park management. However, it is thought that the discussion on the approach proposed in this study can be made more diverse if the analysis is conducted such as the theory of existing urban park use (accessibility, connectivity, etc.) and the spatial analysis related to the current urban park creation and management. It is expected that this point can be studied in the future as well as the park management system.
This study has several limitations. First, the data were limited to five parks in one administrative district due to the Seoul Metropolitan Government’s data export policy. To overcome this limitation, the study results were discussed and verified through a review of prior studies. Future research should include more parks in the analysis. Second, the data were from Spring 2016. Korea has a clear climate that changes seasonally. The difference in the distribution of hot spots over time could be discussed by securing additional data in the future.